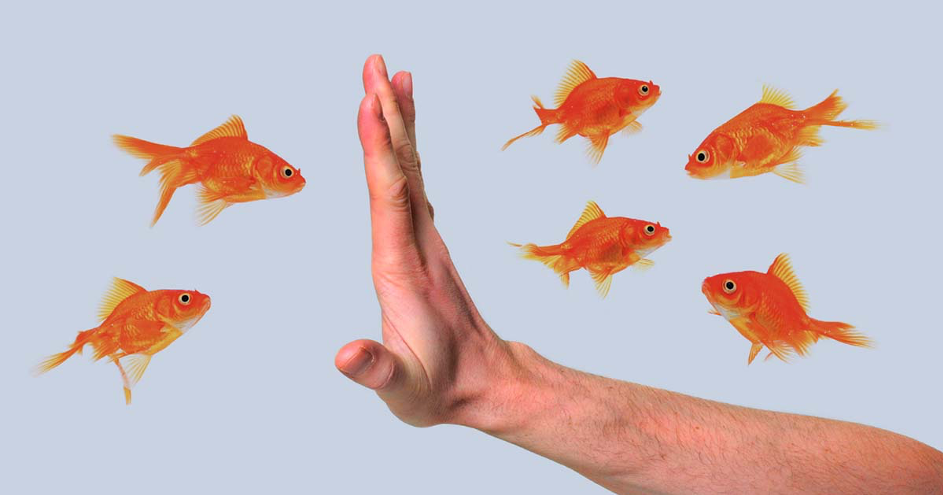
21/03/20
- publications
Algorithms: Biases Control - A Report By The Institut Montaigne
Calculate the shortest route on your phone, automatically create a playlist with your favorite songs, find the most relevant result via a search engine, select CVs that match a job offer: algorithms help you all along the day. But what would happen if a recruitment algorithm was discriminating? Did it systematically leave aside women or ethnic minorities? How do we make sure these errors are highlighted and corrected?
Using more than forty interviews, the Montaigne Institute wishes to provide tangible solutions to limit potential drifts and restore confidence in the algorithms.
This report attempts to give a French perspective to this issue, which today is mainly treated through an American lens. It extends the paper published in 2019 by Télécom Paris and the Abeona Foundation “Algorithms: bias, discrimination and equity”.
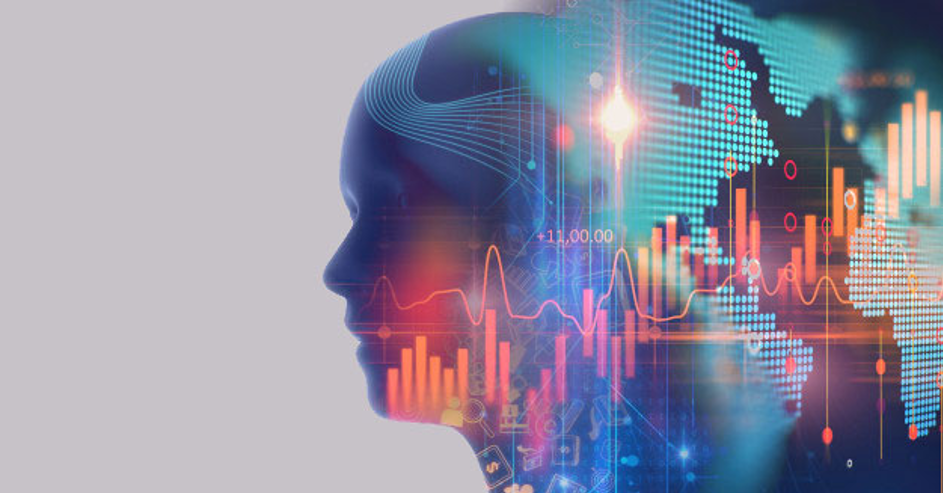
07/12/19
- publications
"Integrating Ethics Into Algorithms Raises Titanic Challenges" (Le Monde)
Two researchers from Télécom Paris, David Bounie and Winston Maxwell, describe for “Le Monde” the tangible solutions to tackle the risks of discrimination that platform algorithms can generate.
More and more examples in justice, health, education and finance, show that artificial intelligence (AI) tools cannot be deployed without control in security systems or access to essential resources. Without these safeguards, they could generate biases, potentially discriminatory, difficult to interpret and for which no explanation is provided to end-users.
The conclusion is becoming increasingly clear: AI must integrate ethics from the design of algorithms. The ethical performance of the algorithm (absence of discrimination, respect for individuals, etc.) must be included in the performance criteria, along with the accuracy of the predictions.
But integrating ethics into algorithms raises titanic challenges, for five reasons: First, ethical and legal standards are often unclear, and do not lend themselves to mathematical formulation […] Second, ethics is not universal [ …] Third, ethics are political […] Fourth, ethics are economic […] Fifth, ethics are temporal.
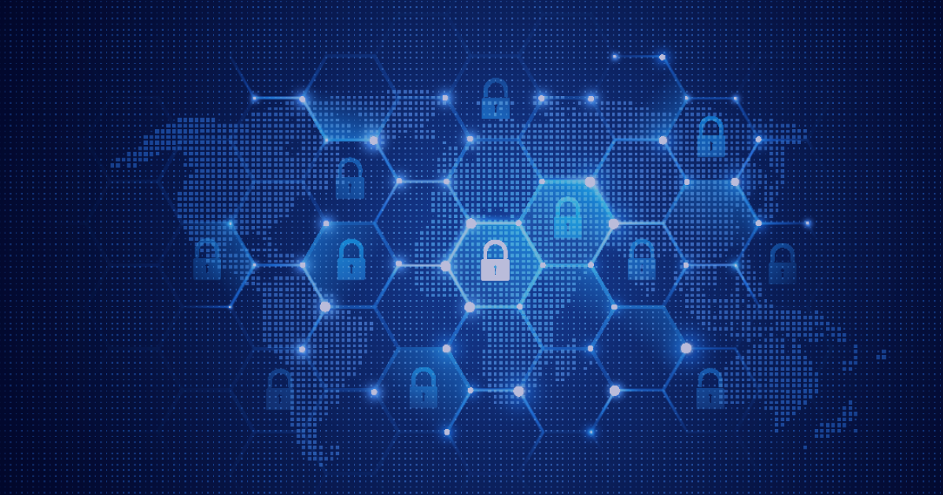
02/09/19
- publications
Is Explainability Of Algorithms A Fundamental Right?
“The demand for transparency on the functioning of algorithms must be addressed with discernment”, assert, in a column to “Le Monde”, researchers David Bounie and Winston Maxwell.
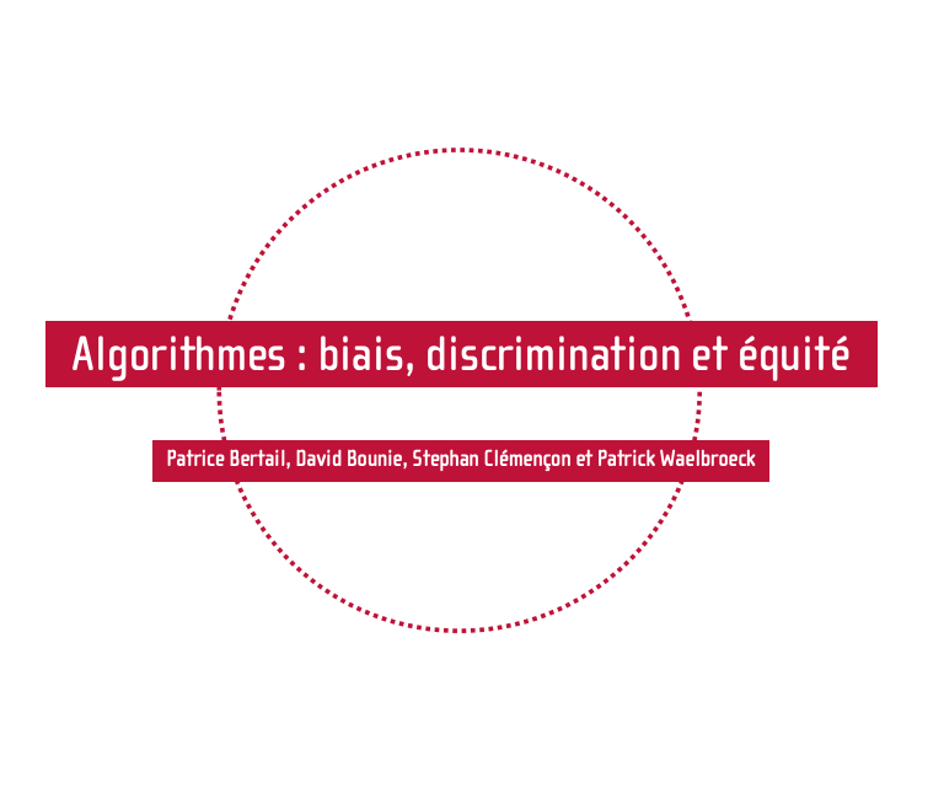
14/02/19
- publications
Algorithms: Biases, Discrimination And Equity
Algorithms are interfering more and more in our daily life like decision support algorithms (recommendation or scoring algorithm) or autonomous algorithms embedded in intelligent machines (autonomous vehicles). Deployed in many sectors and industries for their efficiency, their results are increasingly discussed and disputed. In particular, they are accused of being black boxes and of leading to discriminatory practices linked to gender or ethnic origin. This article aims to describe the biases related to the algorithms and to outline ways to address them. We are particularly interested in the results of algorithms considering equity objectives, and their consequences in terms of discrimination. Three questions motivate this article: By which mechanisms can algorithm biases occur? Can we avoid them? And, finally, can we correct or limit them? In the first part, we describe how a statistical learning algorithm works. In a second part we are interested in the origin of these biases which can be of cognitive, statistical or economic nature. In a third part, we present some promising statistical or algorithmic approaches that can correct biases. We conclude the article by discussing the main societal issues raised by statistical learning algorithms such as interpretability, explainability, transparency, and responsibility.